A Dummy's Guide to Data Science
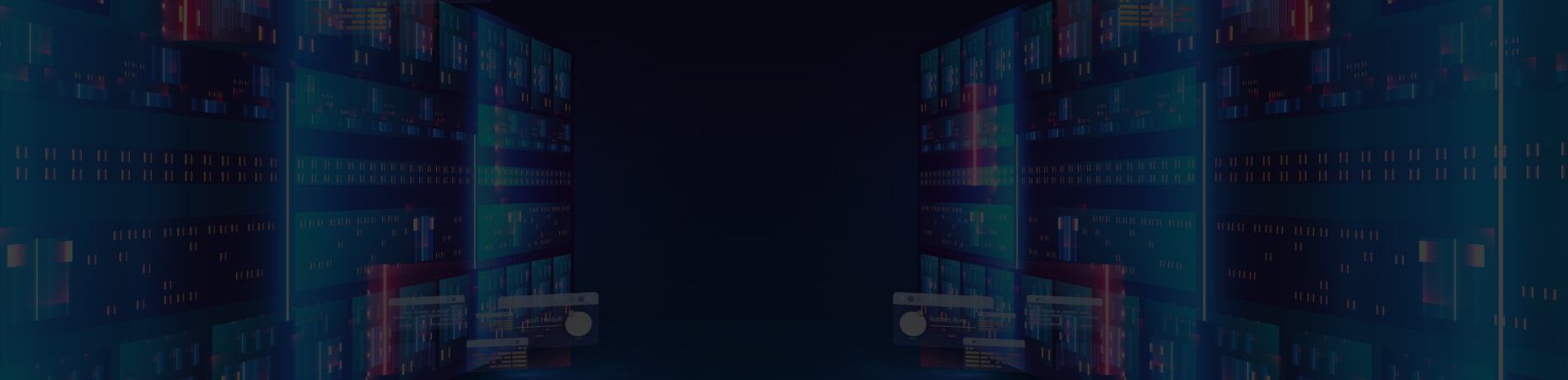
Content Map
More chaptersAccording to Domo Business Cloud, roughly around 2.5 quintillion bytes of data are created every day (add 18 zeros after your 2.5). On average, one person produces at least 1.7MB of data per second. As data is continuously being created, the amount of data that we can access and process is rapidly increasing.
What can we do with this enormous amount of data? How is Data Science changing how we are treating information and reshaping the way we live? If Data Science is an unfamiliar term to you then let’s walk through the basics of Data Science and how this field of study is transforming how enterprises are dealing with data around the world.
What is Data Science?
According to MarketsandMarkets™, the Data Science platform market is expected to hit $322.9 billion by 2026. The 2022 Salary Guide from Robert Half shows that Big Data engineering is now the top paying IT job in the U.S, and Data Science is one of the most sought-after professions. 94% of enterprises agree that data is the core to driving growth, and businesses should utilize their data to better their products and promote innovation. Data Science is now a technology that all business owners should know and take into account to stay relevant in the competitive market.
But first, what is Data Science? Data Science is the integrative approach to extracting deep insights from the vast amount of data for real-world applications through scientific methods, mathematics and statistics, and programming skills. In short, it’s a practice of turning data into solutions. Data scientists prepare data for analysis and processing, including cleansing, aggregating, and manipulating the collected data for advanced data analysis. Data is then presented to analysts and business users to draw insights in the decision-making process for all purposes.
How Does Data Science Work?
Data is now considered the most valuable business asset, the fuel to all business insights. A majority of businesses know that they need Data Science to better their operational processes, yet not everyone fully understands what Data Science is, where it came from, and how it works. Below are the basics of Data Science and what a data scientist does in order to analyze data for actionable insights.
Data Science Foundation
In 1962, John W. Tukey wrote a paper called “The Future of Data Analysis,” which refers to the merging of statistics and computers, the foundation of Data Science. Data Science was first officially introduced as a term in 1974 when Peter Naur proposed it as an alternative way to call computer science in his book “Concise Survey of Computer Methods.” The International Federation of Classification Societies in 1996 was the first conference to feature Data Science as a topic.
Even though Data Science has only emerged recently as a new profession to make sense of the vast stores of Big Data, it is a business field that is complicated yet, at the same time, extremely popular due to its importance for insights and growth. Data Science evolves as a discipline using computer science and statistics to make useful analyses and insights for a wide range of fields, especially astronomy, healthcare, and business intelligence.
What is Big Data?
“Big Data is high-volume, and high-velocity or high-variety information assets that demand cost-effective, innovative forms of information processing that enable enhanced insight, decision making, and process automation.” - Gartner.
Big Data is a term to describe immense structured and unstructured data sets that are generated rapidly at great velocity from a variety of sources. Big Data grows exponentially with time, which means it is hard to manage using traditional data-processing methods.
Since Big Data is too large in volume to be stored and processed in a single computer, other methods are used to collect, store, and extract actionable data from Big Data. Data Science is a domain of study that deals with data using a combination of mathematics and statistics, along with modern tools and techniques to cleanse, prepare, and analyze Big Data to extract insights and information for applications. In conclusion, the ideas of Big Data and Data Science are inseparable.
Data Science Lifecycle
The Data Science lifecycle describes the pipeline that data goes through, from raw data to useful business insights. Data scientists start with identifying the related questions and collecting data from a large range of data sources. The data is then organized and translated into solutions and communicated to the right party for insights and better business decisions. This process includes the following five overlapping and continuing stages:
Capture
After pinpointing the problem that needs to be solved, qualified unstructured and structured data are gathered from all sources via different methods.
Maintain
The raw data is then put relevant data into a consistent format for analytics or Machine Learning or deep learning models to prepare for the data processing step.
Process
After sorting and organizing the right set of data, data is cleaned and examined to use for predictive analytics, Machine Learning, or deep learning algorithms.
Analyze
Data scientists perform different types of analysis to extract insights and gain intuition from the processed data.
Communicate
The result of the data analysis is presented through reports, charts, models, or other visualization methods to give business insights and aid in the business decision-making procedure.
The Rise of Data Science
A study from SINTEF in 2013 revealed that 90% of data in the world was generated within the previous two years. And it didn’t stop then. In 2013, humankind had 2.7 zettabytes of data. By the end of 2020, we had 44 zettabytes of data. Techjury predicted that in 2022 alone, the world would produce and consume 94 zettabytes of data. The amount of data we have is increasing exponentially, and the use of data insights is also on the rise.
In order to make the best use of this enormous amount of data, data scientists have been processing data for better insights in a variety of industries.
Healthcare
Medicine and healthcare are two of the most vital industries to human lives. Did you know that a human body generates two terabytes of data a day, including but not limited to: heart rate, brain wave, stress level, sugar level, and cell generation? To process such a large amount of data to create better health solutions, Data Science is used to collect and analyze related data to figure out the fastest solution to solve even the most formidable health challenges. During the Covid-19 pandemic, Data Science helped a great amount to speed up the treatment and figure out a cure for the deadly contagious disease. By analyzing data related to human mobility, contact tracing, medical imaging, virology, drug screening, bioinformatics, and electronic health records, researchers can gain deep insights into the movement of the virus, how the virus works, how it affects the human body, who are at higher risk of severe symptoms, as well as how to prevent and stop the spread of the coronavirus.
Logistics
Did you know that UPS is saving up to 39 million gallons of fuel and more than 100 million delivery miles each year? Their telemetry program, which began in 2008, is saving the company millions of dollars per year. Their fleet system - On-Road Integrated Optimization and Navigation (ORION) program - costs $1 billion a year and uses Data Science statistical modeling and algorithms to get input based on weather conditions, traffic, construction, etc., with over 250 million data points, along with fleet telematics and advanced algorithms, to improve route optimization. Along with applying Big Data analytics for their routings and shortening the delivery route, they also developed algorithms to predict delivery trucks’ maintenance requirements, which saves UPS millions of dollars in preventing unexpected and unplanned maintenance, which can lead to discontented customers and delays in shipment.
Entertainment
Data Science helps streaming services such as Hulu, Amazon, and Netflix track and analyze what their users watch and enjoy, which then gives insights into what kind of new TV shows and films should be produced. “You do not make a $100 million investment these days without an awful lot of analytics,” said Dave Hastings, Netflix’s director of product analytics. In addition, data-driven algorithms are used to personalize and recommend content the users see based on the users’ viewing history. There are other uses for Data Science, such as forecasting, customized marketing, sentiment analysis, and user segmentation. These applications help entertainment companies optimize their administration procedure regarding budgets, schedules, and plans. Furthermore, Data Science helps collect and examine data to improve customer satisfaction and engagement to create a desirable impact.
Finance
If there’s a field that relies heavily on data, it has to be finance. Data Science and finance go hand in hand, even before Data Science was a thing. Financial institutions were among the first users and adopters of data analytics, especially in risk and fraud detection, along with consumer analytics. Here are the common applications of Data Science in the finance industry:
- Financial Fraud Detection - Frauds are a major concern for all financial institutions. Thanks to the growth in Big Data and analytical tools, financial institutions can now keep track and analyze the unusual patterns in trading data and purchases to improve the algorithms to combat and prevent fraud.
- Risk Management - The most important step of running a successful business is to understand and handle the underlying risks the company must take and overcome. This is the same for finance. Risk management is a field where not only a large amount of data is collected and processed, but it also requires knowledge of math, statistics, and problem solving. Financial institutions usually rely on Machine Learning algorithms to interpret transactions and verify the creditworthiness of customers.
- Real-Time Analytics - Due to the development of dynamic data pipelines and advancements in technology, data can now be processed in real time to keep track of all transactions without any issue of latency. This results in a faster reaction time and minimum delay for financial insights.
- Customer Analytics - Consumers are the core of every business. There is a large amount of customer data that can affect and transform business decisions. By reviewing consumer behaviors and interactions and turning them into useful information and insights, companies can increase cross-sales, measure the lifetime value of a customer, and improve customer engagement.
- Personalized Services - By using speech recognition and natural language processing, financial institutions can provide better interactivity to their users. By evaluating and generating insights with the collected data, financial institutions can optimize their strategies and better their customer services, resulting in increased sales and better customer satisfaction.
- Predictive Analysis - Predictive analysis is one of the most popular applications of Data Science. By applying predictive analysis to finance using deep learning and Machine Learning, businesses can learn and effectively predict future events to help them make a better investment or trading strategy.
- Algorithmic Trading - One of the most crucial aspects of Data Science in finance is algorithmic trading, which allows financial firms to formulate new trading techniques through the use of complex mathematical equations and high-speed computations. A massive amount of data is processed through algorithmic trading and modeled to describe the data streams, which helps financial institutions make better predictions for the upcoming market and the company’s future.
Education
Education is of great importance to society and social good; hence, Data Science is also used to improve the teaching and learning process and enhance the education system. By collecting and studying educational data, educators can conceive and develop a deeper understanding of students’ problems and provide potential solutions, as well as enriching teaching methods and making a significant impact on student motivation.
Another major point that educators have to understand is every student has their own unique way of learning and understanding things. Thus, it is difficult for educational organizations to figure out one method of teaching that can apply to all students. Data Science helps create adaptive learning methods to construct personalized learning experiences for students, which in turn improve student engagement and discover students’ hidden potentials, delivering better educational results.
Technology
As most technologies are developed and evolved through the use of data, whether it is AI, Machine Learning, the internet of things, deep learning, or others, Data Science is the key to technological advancements. Since new technologies are emerging daily and innovation is essential, Data Science proves its worth by collecting and exploring technology trends, learning and adapting to previous errors or mistakes, enhancing and solving potential issues, surveying end users’ opinions and expectations, and optimizing and polishing technology techniques based on processed data and feedback.
Data Science aids in realizing different theories and models and transforms them into knowledge and values to validate and discover patterns, which help scientists design better theories and models for future technology.
Cybersecurity
Cybersecurity is the core of every business, as a lack thereof potentially means the end of the company. Most businesses have a decent amount of stored sensitive customer information, which unfortunately is a big target for cybercriminals. Cyber attacks are very common, and approximately 7 million data records are compromised per day. This increasing risk, combined with the fact that hackers are constantly tweaking their ways and changing their tools and methods to intrude on business systems, makes it harder and harder to catch these online criminals.
Data Science finds patterns that help detect abnormal activities and intrusions before they happen, as well as loopholes in the security systems and predict future attacks, allowing organizations to implement preventive measures and protect business data and information.
Data Science Specializations
Data Science is a broad field that spans numerous industries with increased interest and demand. As businesses have specific goals for Data Science, there are several specializations in Data Science that focus on different aspects, technologies and uses.
Let’s take a look at some of the most important, specialized areas within Data Science right now.
Machine Learning
Machine Learning, at its core, is the study of computer algorithms that can imitate the way that humans learn and adapt through the use of experience and data. Machine Learning is a type of Artificial Intelligence that relies heavily on data in order to teach itself without being programmed to do so.
Data Engineering
Data engineering is the advanced process of getting and storing data from other sources and preparing and transforming raw data into usable data. Data engineers design and build systems used for collecting, storing, and analyzing data for operational uses. The core of data engineering is to gather, clean, and provide the needed data to optimize business performance.
Data Analytics
Data analytics, or Big Data analytics, is a field of Data Science that focuses on extracting actionable insights from Big Data. Data analysis concentrates on answering questions that need answers based on the given data in order to find out valuable insights such as observations, trends, or potential improvements for practical uses.
Business Intelligence
Business intelligence uses techniques such as business analytics, data mining, data visualization, and other data tools to transform data into actionable insights. Business intelligence helps enterprises strategize and optimize their business decision-making procedure to create better data-driven solutions.
Most Important Data Scientist Skills
Without professionals who turn unstructured and structured into actionable insights with the use of cutting-edge technologies, Big Data would mean nothing. However, there are several skills that most data scientists must have in order to bring out the greatest boost to business growth.
Math and Statistics
At the core of Data Science lies statistics. Data scientists use statistics to gather, review, analyze and draw conclusions from data, as well as building models from said data to demonstrate the results. Mathematics and statistics are the two most important concepts of Data Science. Some experts, such as Nate Silver, the founder of FiveThirtyEight, even asserted that Data Science is basically a rebrand of statistics. However, many others disagree as Data Science also heavily involves computer science and domains & business knowledge, besides the use of math and statistics.
Machine Learning
Machine Learning helps automate the processes of Data Science, especially data analysis, and makes data predictions in real time without human intervention. Since the amount of data is enormous and it would take a toll on the data scientists to prepare and extract the data by themselves, Machine Learning makes data scientists’ lives easier by automating the processes through Machine Learning algorithms. This allows data scientists to analyze and examine large chunks of data automatically and make predictions in real time to solve business problems with high accuracy.
Programming
Just as Math and Statistics are a core part of Data Science, computer science is another vital aspect that data scientists must be familiar with. There are several technologies that data scientists, especially data engineers, work with, including but not limited to:
- Hadoop Platform: Hadoop is an open-source software framework for storing giant amounts of data and processing large data sets across clusters of computers through simple programming models. Hadoop is a big must for Data Science as its main function is the storage of Big Data, including both structured and unstructured data. It is basically the first step of Data Science.
- Spark: An open-source, unified, distributed processing system used for working with Big Data. It has a set of libraries for large-scale data processing. Spark runs on memory (RAM) which makes it much faster than driving on disk drives. It is also fully compatible with Hadoop and processes data in real time.
- SQL: SQL - Structured Query Language - is a standard query language for querying and managing relational databases in order to create, maintain, and retrieve data from those databases. It is the most commonly used database system for Data Science.
- Python: A general-purpose programming language and most popular tool for Data Science and analytics. It is also one of the most popular AI programming languages due to its pre-designed libraries that optimize the AI development process.
- R: Supported by the R Core Team and the R Foundation for Statistical Computing, R is a programming language with a wide variety of statistics-related libraries for statistical computing and design.
- SAS: Statistical Analytical System (SAS) is a tool for advanced analytics and complex statistical operations. The main purpose of SAS is to retrieve, report, and analyze statistical data. SAS is especially used for data scientists who are specialized in business intelligence, as it allows users to easily convert data into visuals and graphics, as well as collaborate on interactive dashboards and reports across organizations securely.
- Java: According to Stack Overflow’s 2020 Developer Survey, JavaScript is the most commonly-used language in the world at 69.7%. Even though Python and R are often used more than Java, Java is better for scalability as it makes it easier to scale up or down based on its excellent load balancing features.
- C/C++: While C/C++ is not regularly used for Data Science as most data scientists don’t have a computer science background, and C/C++ requires a fundamental knowledge of programming and is harder to learn and apply compared to declarative languages such as Python, it is extremely fast - C++ is the only language that can process data over a gigabyte within a second!
Data Visualization
Data visualization focuses on presenting information and data in a visual manner, mostly through models, charts, dashboards, or reports. Examples of data visualization concepts for Data Science are:
- Percentages and Lists
- Color Maps
- Interactive Data Displays
- Charts, Graphs, Models
Data visualization is a key tool in displaying a large amount of data, which helps data scientists tell stories and explain insights, highlighting data results with clear visuals. The most effective visualization can help data scientists convey their stories and deliver the right message to decision makers.
Communication & Business Acumen
To be able to solve business issues, one must be familiar with and have a full understanding of how the business works and what it needs to improve. In order to convert data into actionable insights, it is important to have good communication and business acumen skills. Data scientists need to understand the issue at hand, explicate collected data into values, then transform those values into potential solutions and convey them to stakeholders.
Data Science Challenges
With every opportunity comes potential challenges, and it is the same with Data Science. Though Data Science is a must-needed field for every business for growth, it has its own challenges that companies must face and overcome in order to succeed.
Multiple Sources
As businesses are extracting data from multiple sources, the data being collected is not usually in the same format and most likely not always related to a single functionality. Processing this data is a complex operation, and it can be difficult to gather meaningful insights, especially with a large volume of data. Cleansing the data is the most time-consuming procedure of Data Science, especially when the data is unorganized.
The first step to solve this is to make sure to gather the proper data that is useful for business so data scientists do not have to waste time processing unrelated, bad data. Next is to have a centralized platform that allows integration from all data sources and a proper data management plan to prioritize qualified data to save time and effort for both the data scientists and the business.
Data Security
The number one concern of all businesses operating on the cloud is data security. A Clark School study at the University of Maryland shows that there is, on average, a cyber attack every 39 seconds - which means more than 2,200 per day. Cybercrime estimated the cost of cyber attacks globally will reach $10.5 trillion annually by 2025. The most recent huge cyber attack resulted in a $600 million loss on crypto as hackers were able to break into Axie Infinity, a blockchain app game system developed by Sky Mavis, by compromising Ronin, Sky Mavis’ blockchain.
Since Data Science relies heavily on collecting and storing data, mostly confidential data, data security becomes one of the main risks. As hackers are advancing rapidly to keep up with all the newest security tools, it is harder and harder to defend company systems from cyber attacks. Organizations have to install extra checks and utilization for all processes, which also puts a toll on the data scientists in order to access these data.
In order to save time and increase productivity, enterprises have been developing Machine Learning platforms for data security, which aids in safeguarding their data while cutting down on steps needed to authenticate data from verified accounts, as well as training their staff in data security protocols and implementing advanced data security tools.
Unreliable Metrics
One of the many concerns regarding Data Science is the lack of understanding from non-data scientists regarding Data Science metrics. This can lead to unreasonable or undefined KPIs that are unrealistic for the data scientist. Model accuracy changes across different domains and fields; for example, data for A country cannot project results for B country. Just because a chart shows a trend for white shirts does not mean white shirts will be the trend or that the sales of white shirts will go up, for example.
Businesses, especially decision makers, should train themselves and get themselves familiar with Data Science metrics and basic knowledge in order to fully optimize and recognize the analysis. Data should also be categorized and used for the right industry and market. There should also be well-defined metrics to measure the analysis and proper KPIs to analyze the business impact.
Vast Amount of Data
95% of businesses struggle to manage unstructured data, and 73% of data goes unused for analytics purposes. Without a proper pipeline and data processing system, it is close to impossible to process and extract this massive amount of data that is being stockpiled and updated in real time.
As data is massive and might take a long time to be extracted and processed, there comes another concern: out-of-date data. Imagine spending millions of dollars on last year’s analytics or stocking up on products that are out of trend and low in demand. This is especially true in healthcare, where data is vital, and not getting the most updated data might result in “fatal” mistakes.
The most challenging task for every Data Science practitioner is to be able to analyze the accumulated volume of data being generated every second, and that is why the cost of bad or wasted data is probably one of the highest costs for every business. Having the right tools and Machine Learning models for data optimization can speed up the process and save enterprises millions of dollars in costs.
Lack of Tools and Professionals
Due to the fact that Data Science is trending everywhere and driving the industries, everyone is looking for Data Science experts to help their own business. This creates a surge in demand followed by a talent shortage. Despite data scientists being one of the top-paying jobs, companies are struggling to recruit good data scientists for their projects.
Another issue is the lack of skills and tools needed. Most data scientists are specialized in one area, whereas sometimes, they are hired for another area, which they might or might not be qualified for. There is also an experience requirement as Data Science beginners might not have enough understanding to provide correct, suitable, and outstanding advice. Data Science tools might also be costly, which leads to slow data extracting and cleansing processes.
Businesses that are looking into Data Science might consider training their current or new employees on Data Science and invest in the future, or look into alternatives or overseas talent and expand their talent pool.
Communication
to success with business intelligence. In order to optimize Data Science, data scientists have to see eye to eye with the business’ values and strategy. This means both sides have to clearly understand the question they are trying to answer and define the problem and objective before starting the project. The workflow also has to be a result of collaboration with the business stakeholders with proper checklists and areas of concern for proper understanding and identification.
The most important step of a data scientist’s job is to communicate insights to business executives. As management can be unfamiliar with the tools and works behind the models, they solely rely on the data scientists to explain their findings and results. However, as data scientists often have a more technical background, their explanation might not be as persuasive and clear as expected. Essentially, business and IT have to be aligned on the business strategy. Businesses also have to embrace Data Science and understand the true values of data and what they can do to drive business growth.
Cost
“If ‘sexy’ means having rare qualities that are much in demand, data scientists are already there. They are difficult and expensive to hire and, given the very competitive market for their services, difficult to retain.” - Harvard Business Review.
According to a Capgemini report, cost is the biggest Data Science challenge businesses are facing. The average salary for a data scientist in the US is $195,000, making it one of the top-paying jobs worldwide. The high price tag and demand contradict the low supply of highly experienced experts, making it costly for businesses to hire a professional with the right skillset and background.
However, businesses have to understand that in the long run, data can help save them millions of dollars, as well as increasing revenues and bettering customer experiences. While it is indeed costly to start a Data Science department as there is an expert shortage, as well as the number of tools needed, one can turn to other third parties such as outsourcing companies or Data Science services for help temporarily.
What Data Science Brings to Your Business
Data Science is a powerful tool for businesses to utilize and optimize their data. From bettering productivity and customer services, to hiring new candidates and supporting senior staff, to making business-transforming decisions - Data Science has and is helping thousands of businesses around the world study their data to gather valuable insights for analytics and innovation.
Data has been one of the most dominant forces that drive business growth in recent years, and it’s the most important strategic information for companies. Why? Because it ensures that your business is delivering the right product to the right audience. There are many benefits Data Science brings to a business, including but not limited to:
The Future of Data Science
As the amount of data being generated is growing dramatically, Data Science is now needed more than ever to transform data into actionable insights. Data Science is a rapidly expanding field that all enterprises are looking and investing in. Corporations around the globe are adopting Data Science techniques and implementing data technologies for Big Data analytics. A study by NewVantage Partners in 2019 revealed that 97.2% of executives are investing in both Big Data and AI, especially in the financial services industry.
The Big Data analytics market is expected to reach $103 billion by 2023, and poor data quality costs up to $3.1 trillion yearly, just for the U.S. economy alone. This proves that enterprises need to understand the urgent need for Data Science and the possible loss of wasting data potential, and those who have not yet adopted Data Science face the risk of falling out of the competitive market.
The future looks bright for Data Science as data is essential for every business, especially in the finance and medical industries. With the rise of AI, ML, and quantum computing, data scientists will be able to profile and analyze data at higher and higher speeds. Data Science will never fall out of trend as data drives positive changes and speeds up business growth, as well as bettering consumer experiences.
“Data analytics is the future, and the future is NOW! Every mouse click, keyboard button press, swipe, or tap is used to shape business decisions. Everything is about data these days. Data is information, and information is power.” - Radi, data analyst at CENTOGENE
Data Science experts will be increasingly in demand in the future. This means that it is crucial to invest in human potentials today, whether they are juniors or experts, to drive future solutions and implementation. Data scientists will be shaping the future of businesses in the years to come.