Unlocking the Power of Data Science in eCommerce: A Comprehensive Guide
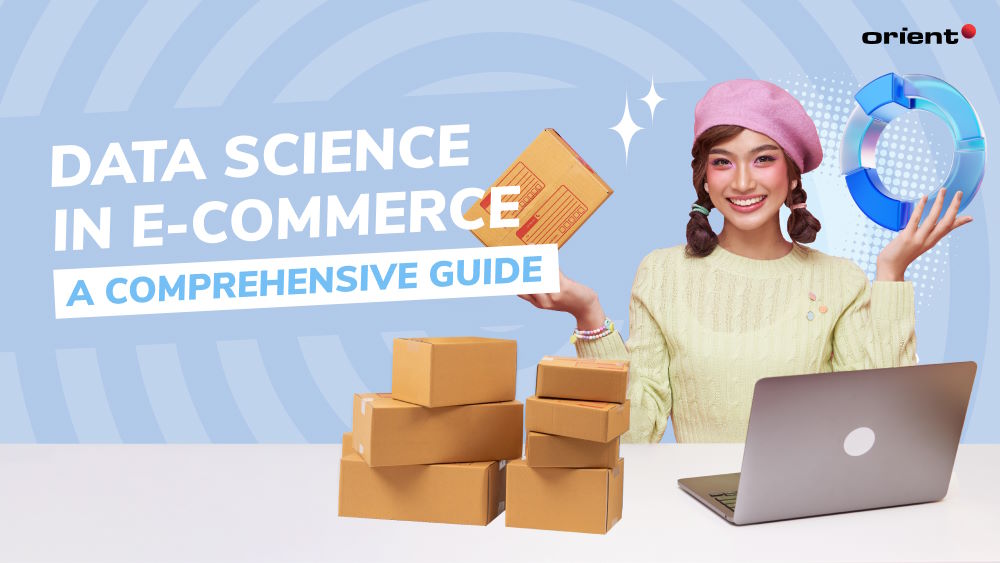
Content Map
More chaptersAs the e-Commerce industry increasingly grows at a rapid pace, businesses are increasingly turning to data science to gain a competitive edge. Data science involves the use of statistical and computational techniques to extract insights from data, and it has become an essential tool for eCommerce businesses looking to understand customer behavior, optimize marketing strategy, and drive growth.
In this comprehensive guide, we’ll explore the applications of data science applications in eCommerce, as well as the techniques and best practices for implementation.
Applications of Data Science in eCommerce
Data science has numerous applications in eCommerce and can provide enterprises with valuable insights into customer behavior, market trends, and operations. Here are some key applications of data science in eCommerce.
Customer Segmentation
Customer segmentation is the act of grouping specific customers based on specific attributes or behaviors that they exhibit. By segmenting customers, businesses can do market basket analysis and personalize their offerings based on the unique needs of different customer groups. Data science plays a crucial role in customer sentiment analysis by analyzing large sets of data to uncover patterns and insights that can be used to identify distinct customer segments.
There are many different ways to segment customers, depending on the specific business goals and the available data. Some common methods of customer segmentation include:
- Demographic segmentation: dividing customers based on demographic characteristics such as age, gender, income, education level, and geographic location.
- Behavioral segmentation: dividing customers based on their behavior, such as their purchase history, website activity, and social media interactions.
- Psychographic segmentation: dividing customers based on their personality traits, values, and lifestyle characteristics.
- Geographic segmentation: dividing customers based on their geographic location, such as country, region, or city.
Data science methodologies can help businesses to segment their customers more effectively by analyzing large sets of customer data and identifying patterns and insights that can be used to create more accurate and targeted customer lifetime value.
Predictive Analytics
Predictive analytics is a powerful tool for eCommerce businesses that allows them to use historical data to make predictions about future events. By analyzing patterns and trends in customer habits, businesses can gain valuable insights into what products customers are likely to buy, when they are likely to buy them, and how much they are likely to spend.
There are several different types of predictive analytics techniques that can be used in eCommerce:
- Regression analysis can be used to predict sales volume based on factors such as price, promotion, and seasonality.
- Decision trees can be used to predict customer behavior based on factors such as product category, price point, and promotional offers.
- Neural networks can be used to predict customer conduct based on a wide range of factors, including purchase records, website activity, and demographic data.
By leveraging predictive analytics, eCommerce businesses can optimize their marketing campaigns, pricing strategies, and inventory management.
Fraud Detection
Fraud is a main concern for eCommerce businesses, and data science may aid in the detection and prevention of fraudulent activities.
Data Science can help eCommerce businesses to detect fraud by analyzing transactional data, such as purchase history, location data, and payment information, and identifying patterns and anomalies that may identify fraudulent activity. By detecting fraud early, businesses can minimize losses and protect their customers’ data and privacy.
Additionally, by using data science to detect and prevent fraud, businesses can create a more secure and trustworthy shopping experience for their customers, which can result in increased customer retention and loyalty.
Inventory Management
Inventory management is the process of managing and tracking a business’s inventory levels to ensure that they have the right products in stock at the right time. Data science can help eCommerce businesses to optimize their inventory management by analyzing sales data, trends, and seasonality patterns to forecast demand and adjust inventory levels accordingly.
By optimizing inventory management, businesses can reduce inventory costs while ensuring that they have sufficient stock to meet customer demand. Additionally, by using data science to optimize inventory, businesses can ensure that they are offering the right products to their customers at the right time, which can lead to increased customer satisfaction and loyalty.
Data Science Techniques for eCommerce
Data science techniques are increasingly being used in the e-Commerce industry to help businesses make informed decisions and gain a competitive edge. Here are some of the key data science techniques for eCommerce and how they can be applied.
Machine Learning
Machine learning is the process of training algorithms to make predictions or decisions based on data analysis. In eCommerce, machine learning can be used to analyze customer behavior and preferences, detect fraudulent transactions, optimize prices and promotions, and improve search results and recommendations.
There are many different types of machine learning algorithms that can be used in eCommerce:
- Supervised learning algorithms can be used to predict customer habits, such as which products a customer is likely to buy next or which customers are most likely to churn.
- Unsupervised learning algorithms can be used to define patterns in customer data, such as product affinities or customer segments.
- Reinforcement learning algorithms can be used to optimize pricing and promotions based on real-time customer conduct.
Natural Language Processing
Natural Language Processing (NLP) is a branch of machine learning that deals with the analysis and generation of human language. In eCommerce, NLP can be used to analyze customer reviews and feedback, improve search results, and automate customer service.
Businesses can use NLP algorithms to analyze customer feedback and identify common issues or complaints. This might assist eCommerce companies in enhancing their goods and services and raising client happiness.
NLP can also be used to improve search results by analyzing customer queries and generating more accurate and relevant search results. Additionally, NLP can be used to automate customer service by analyzing customer inquiries and generating automated responses.
Deep Learning
Deep learning is a subset of machine learning using neural networks to analyze and process complex data. In eCommerce, deep learning can be used to analyze images and videos, improve search results and recommendations, and detect fraudulent transactions.
Deep learning algorithms may be used by businesses to automatically tag product images and identify similar products. This can help businesses to improve their product recommendations and increase sales. Deep learning can also be used to detect fraudulent transactions by analyzing large sets of transactional data and identifying patterns of fraud.
Statistical Analysis
Statistical analysis is the act of using statistical methods to analyze data and draw conclusions. In eCommerce, statistical analysis can be used to analyze customer behavior, optimize pricing and promotions, and predict future trends.
Statistical analysis may be used even by a retail business to determine which marketing initiatives are most successful in boosting sales. This can assist organizations to optimize their marketing spend and create profitable business models.
Statistical analysis can also be used to acquire customers by predicting future trends, such as which products are likely to be popular in the coming months. This might aid businesses in identifying popular products and customer segments, enabling targeted marketing campaigns and improved customer engagement. Additionally, it helps businesses create targeted messages and attract new customers.
Best Practices for Implementing Data Science in eCommerce
Implementing data science in eCommerce can be a complex and challenging process. Still, by following best practices, businesses can ensure that they are maximizing the value of their data and driving meaningful business outcomes.
Developing a Clear Strategy
Before embarking on any data science initiative, eCommerce businesses should develop a clear strategy that outlines their goals, objectives, and desired outcomes. A clear strategy will help businesses to focus their efforts, prioritize their resources, and ensure that their data science efforts are aligned with their business objectives. It is important to involve key stakeholders, such as business leaders and IT professionals, in the strategy development process to ensure that everyone is aligned and on the same page.
Building a Strong Data Infrastructure
To implement data science eCommerce, businesses need to have a strong data infrastructure in place. This includes data storage, processing, and analysis capabilities that can handle large volumes of data and support complex analytics and modeling. Businesses should invest in modern data technologies, such as cloud-based data warehouses and big data analytics platforms, to ensure that their data infrastructure is scalable and flexible.
Hiring the Right Talent
Implementing data science in eCommerce requires skilled professionals from the development team, including data scientists, analysts, engineers, and developers. Businesses should invest in hiring and developing the right talent to support their data science initiatives. This includes identifying the necessary skills and expertise required for each role, as well as providing ongoing training and professional development chances to keep up with the latest software development technologies in the field.
Continuously Monitoring and Evaluating
Implementing data science in eCommerce is an ongoing process that requires continuous monitoring and evaluation. Businesses should establish metrics and KPIs to measure the success of their data science initiatives and regularly review and adjust their strategies based on the results. Additionally, businesses should stay up-to-date on emerging trends and technologies in the field and be willing to experiment and innovate to stay ahead of the curve.
Conclusion
In conclusion, data science is playing an increasingly important role in eCommerce, and businesses that embrace data science are better positioned to compete and succeed in today’s digital marketplace.
If you are a business looking to embrace data science eCommerce, we encourage you to consider Orient Software as your outsourcing partner. Contact us today to learn more about how we can help you unlock the power of data science and drive growth and innovation in your eCommerce business.