
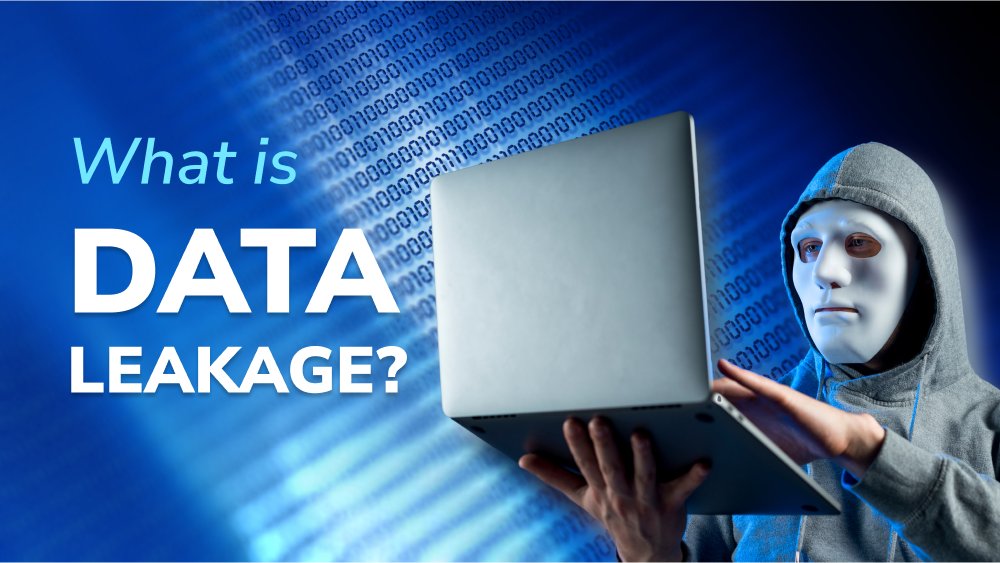
What Is Data Leakage? The Silent Assassin Can Destroy Your Business
Data leakage can have serious consequences for businesses of all sizes. What is data leakage? How do you prevent it from happening to your organization?
Artificial intelligence has made tremendous progress in recent years, but systems still struggle with tasks they have not been explicitly programmed for. Researchers are investigating new techniques to help AI become more adaptive and capable of learning from limited data, like humans. One promising approach is known as meta learning or learning to learn. But what is meta learning?
Meta learning aims to develop machine learning models that can generalize skills and knowledge gained from one task to rapidly learn new related tasks. Did you know the human brain exhibits strong meta learning abilities that allow us to recognize novel visual patterns after seeing just a few examples? Inspired by this, scientists seek to endow AI with similar capacities through methods like model parameter optimization and example weighting.
This article explores the concepts and applications of meta learning, an emerging field at the forefront of advanced AI development. It will discuss research demonstrating how neural networks trained with meta learning can match or exceed human-level performance on challenging few-shot learning problems. The potential real-world impact of more human-like, sample-efficient machine learning will also be examined.
Meta learning, also known as “learning to learn”, is a learning process enhancement of the AI. It’s the difference between teaching a student facts and teaching them how to study effectively. This technique makes AI to become faster and more flexible to undertake other tasks with limited information and even when initialized in different context.
This brings us to the next fact that meta learning is based on how human beings learn. Like the professionals sharpening their problem-solving expertise, the meta learning algorithm reinforces its learning experiences. This means that AI can learn new things as the field progresses, thus being very useful where things are constantly changing, such as in robotics, health, and finance.
In conventional machine learning and deep learning, a model is developed from a big data set to improve the model’s performance for a particular task. The model parameters are adjusted during training and through an optimization-based meta learning process with different methods, such as gradient descent. However, this approach can be problematic, especially when the model is faced with new tasks that differ significantly from the training set. Thus, meta learning can overcome this problem as it is based upon the process of learning. Unlike other models, such as a ‘task-specific’ model, a meta learning model is designed to learn the learning process so that it can learn new tasks with little data quickly.
Meta learning operates through a systematic process designed to teach algorithms how to learn more effectively from previous experiences. This process involves several key steps that enable models to adapt quickly to new tasks with minimal data.
The meta learning process typically begins with the collection of multiple tasks or datasets, which serve as the foundation for developing a meta-learning model. The first step involves defining a set of tasks that are related but distinct, allowing the model to learn from various contexts and conditions. Each task is usually represented by its own dataset, complete with training and testing subsets.
Following that, the model undergoes a training phase where it is trained through multiple learning episodes. In this stage, the algorithm is trained with the training data for each given task to find the optimal values for its parameters. Importantly, the model does not solely predict how to complete each task on its own; rather, it discovers how various tasks are similar to and distinct from one another so that it can apply that knowledge to future tasks.
After the training phase, we assess the model’s performance based on its generalization capabilities on new, unseen tasks. This is often done by further training the model on a few instances from the new task as evidence of the model’s quick adaptation ability. The effectiveness of transfer learning is then evaluated based on the overall accuracy and efficiency the model achieves in the new task.
Meta learning algorithms are designed to extract useful information from past learning experiences, allowing them to generalize across different tasks. They accomplish this by using techniques that allow them to grasp how their learning approaches must change in view of the data they encounter.
A major part of this learning process is the concept of a meta-learner, which operates at a higher order than the standard learner. The meta-learner evaluates the outcome of the base learner in various tasks and determines which of the approaches would achieve the best outcome. In that way, it derives a set of heuristics or rules that guide how the base learner should create/get its strategy when confronted with new tasks.
That is why the learning-to-learn mechanism is especially effective when data is scarce. It is more efficient than other machine learning methods, which would demand the model undergo new training from scratch and instead allow the model to build on what it has learned. This means that the model is capable of adjusting to new conditions quite easily, and this is a serious advantage in terms of requirements for time and data for training.
There are several types of meta learning approaches, which vary in the way they enable fast adaptation and learning.
Model-Agnostic Meta Learning (MAML) is one of the most famous approaches, and it is designed to train models in a way that allows them to complete new tasks with just a few gradient updates. This model-based meta learning type operates by optimizing the initial parameters of a model to be in a state that is conducive to quick learning. During training, the model is exposed to a variety of tasks, and the optimization process focuses on ensuring that a small number of updates can lead to good performance on new tasks. This technique is particularly effective in environments where rapid adaptation is crucial.
Few-Shot Learning is another important area within meta learning, designed to enable models to perform well with very few examples from a new task. This approach is particularly relevant in applications where data collection is expensive or impractical. Few-shot learning algorithms apply the learned information from prior tasks to infer the new tasks, thus making good assumptions from limited data. Common techniques being used in few-shot learning include prototypical networks, Siamese networks, and metric-based meta-learning for creating embedding representations that allow comparison of new examples with learned prototypes.
Gradient-Based Meta Learning encompasses various methods that utilize gradient descent techniques to optimize learning across tasks. This approach typically involves algorithms that adjust the base learner’s parameters based on the gradients computed from multiple tasks. Through the observation of how these gradients vary over different tasks, these algorithms can then determine methods of learning that improve performance in new scenarios.
There are also related approaches, such as meta-reinforcement learning, where the model uses rewards from other tasks to make decisions. This makes the performance of the meta learning models better with each use since it learns from the results of its decisions.
Meta learning has been successfully applied in various fields, which confirms its effectiveness and versatility.
Healthcare: In the healthcare sector, different meta learning methods are useful in increasing diagnostic precision and making management suggestions. For example, meta-learning algorithms can help to process a patient’s data from various sources, including EHR, medical imaging, and genomics, to come up with treatment strategies. One notable use is in predicting the response of patients to certain drugs, where meta learning aids clinicians in changing the drugs used based on past experiences with similar patients. This approach not only improves the quality of the patient’s therapeutic results but also minimizes the number of attempts required to prescribe drugs.
Finance: Meta learning has also been applied to the finance industry specifically to improve predictive modeling for risk and fraud analysis. Meta learning algorithms are then applied by financial institutions to analyze the transaction data with the aim of detecting any discrepancies that may be associated with fraudulent activities. These models are capable of identifying past fraud trends in various transactions and are also easily adaptable to changed fraud strategies, thus enhancing the detection rates and the reduction of losses. Furthermore, meta learning can enhance credit scoring models, as they can make generalizations from a number of datasets, making better evaluations of creditworthiness.
Autonomous Vehicles: Meta learning is used in self-driving cars to enhance decision-making skills and flexibility in challenging situations. For instance, self-driving cars must be able to maneuver through different terrains and road conditions and avoid objects on the road. With this approach, self-driving cars and other autonomous vehicles can effectively and rather swiftly learn their conduct based on certain experiences of nature that have occurred in the past. This capability increases reliability and performance; the self-governed system can manage novel conditions, such as altered road conditions or weather.
With the advancement in the technology sector becoming apparent, the possibility of meta learning in providing flexibility and effectiveness in numerous fields is seen more evidently. We at Orient Software embrace the continuous improvement of our status as the leading machine learning developers and can attest to the potential of meta learning for your enterprise.
No matter the industry you belong to (healthcare, finance, robotics, or any other sphere), our team of dedicated experts is always prepared to assist you in exploring the potential of meta learning with references to your business. Our company has delivered many AI projects across the globe that help businesses remain relevant and make the right analyses in today’s complex world.
Do not pass the opportunity to develop your business by incorporating advanced technologies. Now is the high time to contact Orient Software so as to know how we can help you implement meta learning strategies that can make you unique in your field. So let’s realize the full value of your data and create the path for a future that is faster and smarter.
Data leakage can have serious consequences for businesses of all sizes. What is data leakage? How do you prevent it from happening to your organization?
Within the frame of this article, let’s talk about the benefits of going for artificial intelligence outsourcing services. Let’s get started.
AI apps for Android can do much more than just create content. Check out these ten best AI applications for a better quality of life.
Have you ever wondered how AI in fintech can make your money work smarter for you? Discover the transformative power of AI in our latest article.
The debate between AI vs. human brains has long been an endless topic in society. Human intelligence currently surpasses AI, but will it last?